Computer Science & IT
Coding & Algorithms Development
- Shrewd Object Visualization Mechanism
- Robotic Process Automation
- Role of AI in Healthcare
- Natural Language Processing
- Edge Computing
- AI For Cybersecurity and Knowledge Breach
- Reinforcement Learning
- Machine Learning in Hyperautomation
- The Intersection of ML and IoT
- Consistent Integration with Other Languages
Edge Computing
Edge computing is changing the way data is being handled, processed, and delivered from various devices all over the world. The IoT along with those applications that require real-time computing power drive edge-computing systems. Faster networking technologies allow for edge computing systems to support or create real-time applications such as self-driving cars and robotics. According to Gartner, in edge computing, information processing is positioned close to the edge from where people or things consume or produce that information. Thus, edge computing brings data storage closer to the devices where it is being gathered. Thus, the data does not undergo any latency issues that can possibly affect the performance of an application. Moreover, companies can save money by having the processing done locally. When the number of devices transmitting data simultaneously grows, there will be problems in terms of quality and cost. Edge-computing services help solve these problems by being a local source of processing and storage for most of the systems.
Cost saving is the main driver towards installing an edge-computing design. Next biggest benefit of edge computing is its ability to process and store data faster, thereby enabling more efficient real-time applications that are critical to establishments. With improved interconnectivity and edge access to more core applications, edge computing is certain to be one of the main advanced devices in the server and storage market in the coming years.
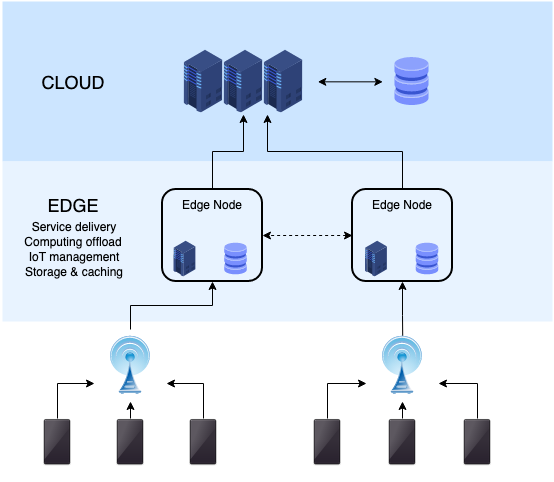
Source: en.wikipedia.org
References
- Wang, X., Han, Y., Leung, V. C., Niyato, D., Yan, X., & Chen, X. (2020). Convergence of edge computing and deep learning: A comprehensive survey. IEEE Communications Surveys & Tutorials, 22(2), 869-904.
- Pustokhina, I. V., Pustokhin, D. A., Gupta, D., Khanna, A., Shankar, K., & Nguyen, G. N. (2020). An effective training scheme for deep neural network in edge computing enabled Internet of medical things (IoMT) systems. IEEE Access, 8, 107112-107123.